FDA Issues Draft Guidance On The Use Of AI To Support Regulatory Decision-Making For Drug And Biological Products
By Susan Shockey, Clarkston Consulting
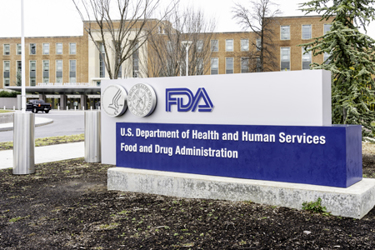
The U.S. FDA issued a draft guidance, Considerations for the Use of Artificial Intelligence to Support Regulatory Decision-Making for Drug and Biological Products, on January 6, 2025.1
The FDA stated in the federal register announcement2 that it recognizes that the use of artificial intelligence (AI) in drug development is broad and rapidly evolving. This guidance proposes a risk-based credibility assessment framework relating to AI technologies, the data used to train the technologies, and governance around the technologies that may be used for establishing and evaluating the credibility of an AI model for a particular context of use (COU). When finalized, this guidance is expected to help ensure that AI models used to support regulatory decision-making are sufficiently credible for the COU.
Background: The Impetus For This Draft Guidance
Continuous advancements in AI hold the potential to accelerate the development of safe and effective drugs and enhance patient care. The increasing use of AI for information used to support regulatory decision-making may positively impact a number of critical analyses, for example:
- reducing the number of animal-based pharmacokinetic, pharmacodynamic, and toxicologic studies
- using predictive modeling for clinical pharmacokinetics and/or exposure-response analyses
- integrating data from various sources to improve understanding
- analyzing large sets of data for the development of clinical trial endpoints or assessment of outcomes
- processing reports for adverse drug information.
The use of AI in these areas also presents some unique challenges. The variability in the quality, size, and representation of datasets for training AI models may introduce bias and raise questions about the reliability of AI-driven results. AI models are quite complex, and understanding how they are developed and how they arrive at their conclusions may be difficult — which necessitates methodological transparency in submissions. Another challenge is the potential for some AI models’ performance to change when new data inputs are introduced and these inputs differ from the data on which the model was trained (i.e., data drift). To address this challenge, a set of planned activities to ensure the model’s performance, called life cycle maintenance, is required.
The New Draft Guidance
This draft guidance is intended to address these concerns and provide recommendations to assist sponsors in their use of AI for regulatory decision-making regarding safety, efficacy, or quality in drug and biological product submissions. It addresses three main topics:
- A risk-based credibility assessment framework for AI use in the drug product life cycle.
- Special consideration: life cycle maintenance of the credibility of AI model outputs in certain contexts of use
- Early engagement with the FDA, especially when the sponsor is uncertain if their use of AI is within the scope of this guidance.
Note: The guidance does not apply to the use of AI models in drug discovery, or when used for operational efficiencies, such as internal workflows or resource allocation, or any other models that are not related to regulatory decision-making.
A Risk-Based Credibility Assessment Framework
To establish and assess the credibility of an AI model output for a specific COU, the guidance provides a risk-based credibility assessment framework consisting of a seven-step process based on model risk:
- Define the question of interest that will be addressed by the AI model.
The first step in the framework is to define the question of interest. The question of interest should describe the specific question, decision, or concern being addressed by the AI model. A variety of evidentiary sources may be used to answer the question of interest.
- Define the Context of Use (COU) for the AI model.
The COU should describe in detail what will be modeled and how model outputs will be used. The COU should also include a statement on whether other information (e.g., animal or clinical studies) will be used in conjunction with the model output to answer the question of interest.
- Assess the AI model risk.
Model risk is a combination of two factors: (a) model influence, which is the contribution of the evidence derived from the AI model relative to other contributing evidence, and (b) decision consequence, which describes the significance of an adverse outcome resulting from an incorrect decision. Assessing model risk is important because the credibility assessment should be commensurate with the AI model risk and tailored to the specific COU.
- Develop a plan to establish the credibility of AI model output within the COU.
The sponsor’s credibility assessment plan may be submitted to the FDA for early consultation and should include the sponsor’s proposed credibility assessment activities based on the question of interest, COU, and model risk. This would include a description of the model and its development process, how the development datasets were determined to be relevant and reliable, the model training process, and the model evaluation process to assess the adequacy of the model’s performance.
- Execute the plan.
This step involves executing the credibility assessment plan. As discussed in step 4, discussing the plan with the FDA prior to execution may help set expectations regarding the appropriate credibility assessment activities for the proposed model based on model risk and COU, and also to identify potential challenges and how such challenges can be addressed.
- Document the results of the credibility assessment plan and discuss deviations from the plan.
The results of the credibility assessment plan are documented in the credibility assessment report, which is intended to provide information that establishes the credibility of the AI model for the COU. This is generally created during the execution of the credibility assessment plan and includes a description of the results from steps 1 through 4, including any deviations.
- Determine the adequacy of the AI model for the COU.
Based on the results documented in the credibility assessment report, either the sponsor or the FDA will determine if the model credibility is sufficiently established for the model risk. Several options are available if the model is not appropriate for the COU, including incorporating additional evidence and data, adding appropriate controls to mitigate risk, or modifying the modeling approach.
Life Cycle Maintenance
Life cycle maintenance of the credibility of AI models is a set of planned activities to continuously assess an AI model to ensure the model’s performance and its suitability throughout its life cycle for the COU. This includes assessment of additional data being input, continuous monitoring of model output and accuracy, identifying potential issues, and taking corrective actions like retraining or re-tuning the model, as necessary. A risk-based approach for life cycle maintenance may help sponsors assess the impact of any changes to the AI model performance.
Early Engagement with the FDA
The FDA strongly encourages sponsors and other interested parties to engage early with the FDA in order to set expectations regarding the appropriate credibility assessment activities for the proposed model based on risk and COU. Early engagement also will help sponsors identify potential challenges and determine how such challenges may be addressed.
In addition to the standard formal meeting request, the guidance includes an extensive table providing a list of other engagement options for sponsors.
Request for Public Comments by April 7, 2025
Because these new recommendations may have a substantial impact, the FDA has issued this as a draft guidance, with a request for comments from the public by April 7, 2025. In particular, the FDA is asking for feedback on how well this draft guidance aligns with industry experience and whether the options available for sponsors and other interested parties to engage with the FDA on the use of AI are sufficient. The agency will review and consider comments received before finalizing this guidance.
Comments may be submitted at any time with reference to Docket No. FDA-2024-D-4689 here.
References
- U.S. Food & Drug Administration. (2025, January). Considerations for the Use of Artificial Intelligence To Support Regulatory Decision-Making for Drug and Biological Products. https://www.fda.gov/regulatory-information/search-fda-guidance-documents/considerations-use-artificial-intelligence-support-regulatory-decision-making-drug-and-biological
- Federal Register. (2025, January 7). Considerations for the Use of Artificial Intelligence To Support Regulatory Decision-Making for Drug and Biological Products; Draft Guidance for Industry; Availability; Comment. https://www.federalregister.gov/documents/2025/01/07/2024-31542/considerations-for-the-use-of-artificial-intelligence-to-support-regulatory-decision-making-for-drug
About The Author:
Susan Shockey is a director with Clarkston Consulting and has wide-ranging experience in quality and regulatory compliance. She has 18 years in the life sciences area, focusing on quality systems, quality process improvement, and inspection preparation and remediation. Prior to that, she spent 15 years in quality assurance engineering supporting manufacturing, testing, and validation of NASA space flight and military hardware.